It is over a decade ago that Richard Florida introduced the “rise of the creative class”. If decaying cities wanted to survive, they had to open cool bars, shabby-chic coffee shops, and art venues that attract young, educated, and tolerant residents. Eventually, the mysterious alchemy of the creative economy would build a new and prosperous urban core. In 2016, Florida admitted in his book ‘The New Urban Crisis’ he was wrong. The rise of the creative class in places like New York, London, and San Francisco created economic growth only for the already rich, displacing the poor and working classes. “Much more than a crisis of cities,” Florida writes, “the New Urban Crisis is the central crisis of our time” — “a crisis of the suburbs, of urbanization itself and of contemporary capitalism writ large.”{1}
The crisis Florida describes is in a lot of ways connected to the process of gentrification. As scholar Sarah Schulman states: “Gentrification is a process that hides the apparatus of domination from the dominant themselves. (…) Physically it is an urban phenomenon: the removal of communities of diverse classes, ethnicities, races, sexualities, languages, and points of view from the central neighborhoods of cities, and their replacement by more homogenized groups.”{2}
Since research about gentrification predominantly focuses on socio-economic developments, it is our intention to focus on the visual effects of this phenomenon. So how do we relate this development to aesthetics of these bars, shops, and other (semi) public spaces?
Philip Kotler was one of the first scholars to recognise a store’s atmosphere as one of the most significant features contributing to its success with customers. In ‘Atmospherics as a Marketing Tool’ he concludes that store design is a type of “silent language” to which little attention has been given in the past.{3} Kotler writes that atmosphere first serves as an attention-creating medium. This means that certain visual elements such as colour and shape stand out and attract the attention of a potential customer. Secondly, the atmosphere serves as a message-creating medium, meaning that the visuals imply what the store’s style of merchandise and target clientele is. Thirdly, the atmosphere serves as an affect-creating medium, meaning that the visual qualities intentionally arouse a positive feeling towards or in the store and thus increase the chance that a customer will purchase an item.{4}
One of the these globally erupting styles where Kotler’s theory would apply has recently been described by different journalists, designers and scholars as “AirSpace”: “It’s marked by an easily recognisable mix of symbols – like reclaimed wood, Edison bulbs, and refurbished industrial lighting – that’s meant to provide familiar, comforting surroundings for a wealthy, mobile elite, who want to feel like they’re visiting somewhere “authentic” while they travel, but who actually just crave more of the same: more rustic interiors and sans-serif logos and splashes of cliché accent colours on rugs and walls.” In recent years, Airspace has been spreading across the world, from Bangkok to Beijing, Seoul to San Francisco. Everywhere you go, hip and seemingly unique spaces all look the same.
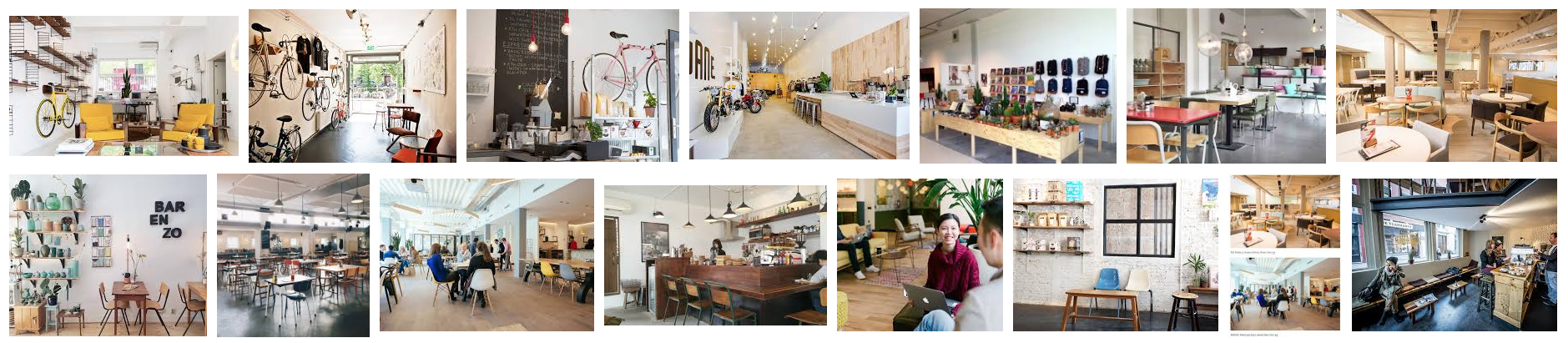
“We could call this strange geography created by technology, AirSpace (…) the realm of space that share the same hallmarks everywhere you go: a profusion of symbols of comfort and quality, at least to a certain connoisseurial mindset. Minimalist furniture. Craft beer and avocado toast. Reclaimed wood. Industrial lighting. Cortados. Fast internet. The homogeneity of these spaces means that traveling between them is frictionless. Changing places can be as painless as reloading a website. You might not even realize you’re not where you started.”{5} It is a concept that is introduced from our ‘virtual world’ to describe a phenomenon that is happening within our ‘physical world’.
What does the spread of Airspace tell us? Its problematic aesthetics are often described as a highly personal expression of taste. If you don’t like it, avoid it. Yet some would argue AirSpace creates a division between those who belong in the slick, interchangeable places and those who do not. Access to AirSpace is expensive, whether it is the amount you pay for a coffee in a bar or the rent/membership for a WeWork office space: if you can’t afford it, you are shut out. One wonders, is “form follows profit (…) the aesthetic principle of our times”?{6}
Yet it is not only the formal question whether you have money enough that is deemed important. ‘Access’ is in this sense also determined by whether you ‘belong’ in the particular space. Do you have the right clothing, ethnic background, age, or other characteristics to fit in?
In her research From Warehouse to Wonderland. About Visualising Retail Gentrification in North-Brooklyn Elise Vleugels summarizes this effect: If you understand it (the store) and can afford it (the merchandise), then come on in, you’re part of the group.{7} Vleugels concluded there is a conscientious crafting of messages onto stores’ exterior facades that communicate the store’s intended distinctiveness and creative attitude.{8} This means that the cultural symbols used in store design not only reflect the personality, taste, and lifestyle of the new owners and clientele but also throw up subtle barriers between them and people who do not understand this visual language.{9}
This not only affects the interior of these bars and shops, but also the exterior of these spaces. This phenomenon could be termed “street gentrification”, a phrase encompassing all transformations in the realm of non-domestic or public areas which are visible as one walks down a sidewalk and tangible features of growing neighbourhood vitality that are encountered as part of everyday life.{10}
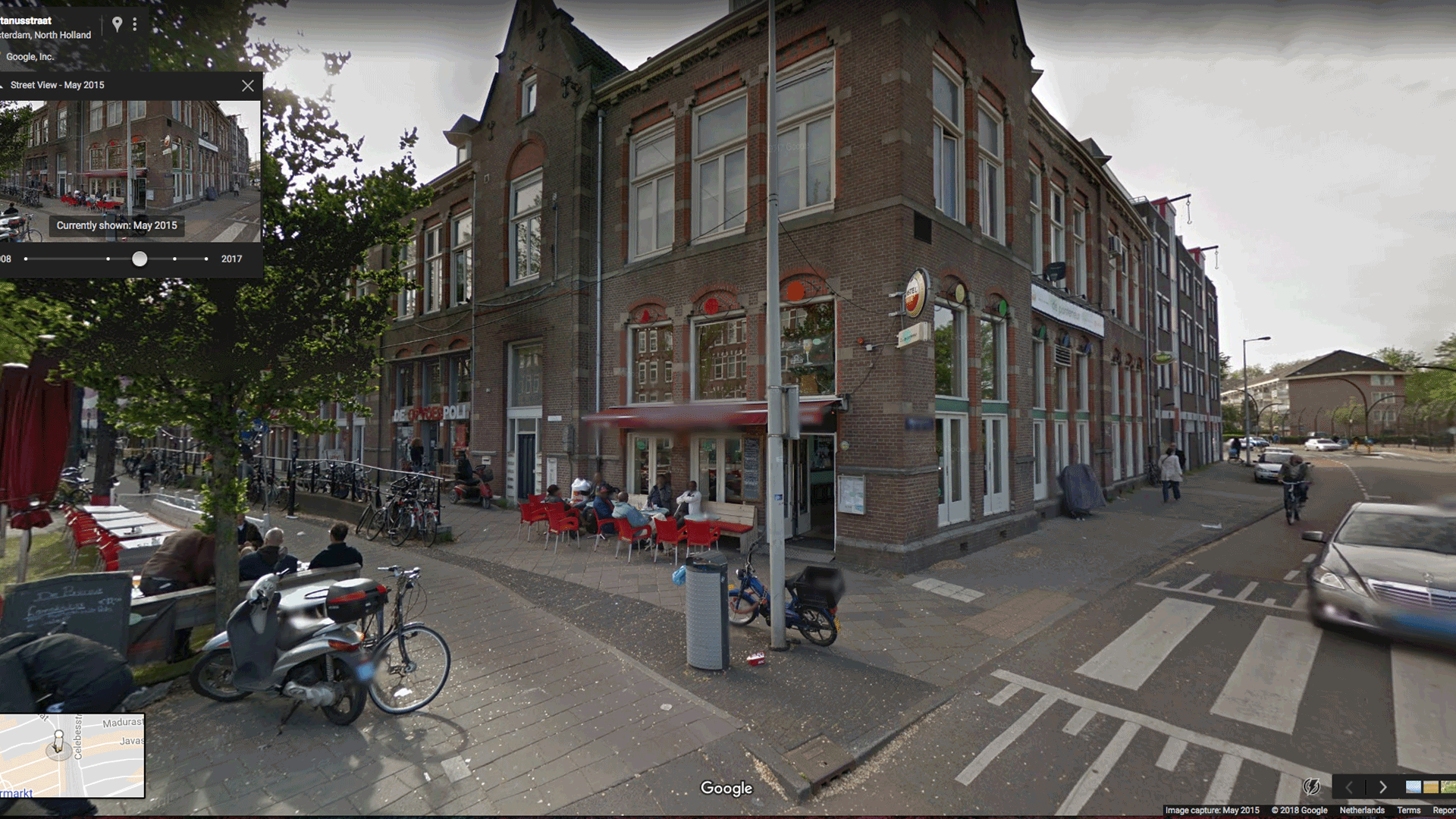
Furthermore, there is a peculiar interaction between aesthetics and modern-day technology that seem to amplify each other. Platforms such as Instagram, Foursquare and Airbnb are making it easier to switch ‘seamlessly’ between different places, but also seem to provoke an aesthetical style that users recognize and feel they ‘belong’ to.
The effects of barriers in society
To what degree is it a problem that these kinds of aesthetical styles create barriers between different groups of inhabitants of cities?
Richard Sennet describes cities are becoming closed systems where people from different social, racial, and economic backgrounds avoid interacting with each other. According to Sennet, cities should instead be built in a way that encourages people to meet more often and empower each other instead of magnifying their differences. This way one creates open spaces where different groups meet and anything is possible.{11}
In his influential book The Great Good Place, Ray Oldenburg (1989, 1991) introduces the concept of a ‘third place’. In community building, the third place (or third space) is the social surroundings separate from the two usual social environments of home ("first place") and the office ("second place"). Examples of third places would be environments such as cafes, clubs, public libraries, or parks. Oldenburg argues that third places are important for civil society, democracy, civic engagement, and establishing feelings of a sense of place.{12}
This relates to the concept which Robert Putnam called social capital: "connections among individuals - social networks and the norms of reciprocity and trustworthiness that arise from them."{13} Social capital can be found in friendship networks, neighbourhoods, churches, schools, bridge clubs, civic associations, and even bars. According to Putnam and his followers, social capital is a key component to building and maintaining democracy. Putnam believes that social capital can be measured by the amount of trust and "reciprocity" in a community or between individuals. Putman speaks of two ways of looking at social capital. The first one, bonding in social capital is referred to as social networks between homogenous groups. The second, bridging in social capital is referred to as social networks between socially heterogeneous groups, allowing different groups to share and exchange information, ideas, and innovation and builds consensus among the groups representing diverse interests.{14} Could you argue that gentrification and aesthetical styles that cohere with it create social capital that is ‘bonding’ between homogenous groups, but fails to ‘bridge’, subsequently even creating a bigger gap between socially divided groups?
Airspace has an interesting link with another concept that finds its origin within the digital world, the notion of the filter bubble, where “we will increasingly be typed and fed only news that is pleasant, familiar, and confirms our beliefs-and because these filters are invisible, we won't know what is being hidden from us. Our past interests will determine what we are exposed to in the future, leaving less room for the unexpected encounters that spark creativity, innovation, and the democratic exchange of ideas.”{15} We would argue that gentrification processes and the aesthetical styles that cohere with it, such as AirSpace, often function as offline-versions of a filter bubble, avoiding serendipity. What is recommended on services Spotify and Netflix, shows in our feed on Facebook and Instagram and is offered through Foursquare does not only resemble the way we are moving through our city, but is also influencing it.
Gentrification not only manifests itself in a certain aesthetical style that is easy to recognize but it also fulfils a function. It creates a division to the groups that ‘get’ and ‘belong’ in these particular spaces and those who do not. Rather than only speaking of a specific style it is a marker that determines in what way people live together in a city. And these markers do influence how groups of different backgrounds interact each with other and consequently affect the way our civil society functions. The strange interaction between technology and aesthetics makes it possible to switch ‘seamlessly’ between different spaces, avoid interaction and therefore create the ‘closed systems’ Sennet describes, or physical versions of the ‘filter bubbles’.
Using computer vision to research archives
Convolutional Neural Networks (CNN) are part of computer vision studies: a field which is concerned with using computers to gain a high-level understanding of images. It relies on mathematical techniques to recover the shape and appearance of objects in imagery. Since the late 1980s, several methods have been developed to detect shapes in images that represent specific objects, which could subsequently be used to categorize these objects. Object recognition and object classification are two of the most important tasks that researchers hoped to teach computers. Early computer vision techniques looked for specific, predetermined, combinations of shapes.{16} CNN’s have been successful in identifying faces, objects, and traffic signs apart from powering vision in robots and self-driving cars.{17}
How does a CNN work? First of all, algorithms do not “look” at an image in the same way as humans, but they are inputted matrices of pixel values. For a computer vision algorithm, each image consists of a grid of numbers between 0 and 255 that contain information on how dark the pixels are. Together these pixels display a specific hierarchy or conceptual structure: a cat, for example. In the end, computer vision algorithms are designed to detect these high-level features of the image.{18}
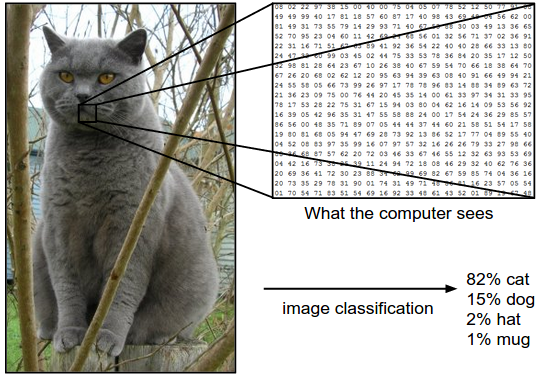
The main challenge here is the fact that an image can show a cat in many different positions. The cat can be walking, sitting down, or climbing up the stairs. Furthermore, specific parts of the cat, such as its head or trail, might be missing from the image. A CNN can recognize all these different “possibilities” of a cat, by looking at multiple lower-level features of an image that uniquely predict the presence of a cat in a wide array of possible positions. A computer vision algoritme sees these low-level features as spatial relationships between a group of pixels in the larger grid of pixels.{20}
The neural network extracts these low-level features from images using a mathematical process called a convolution. A convolution changes the value of a particular pixel on the basis of the values of the pixels that surround it. As a result, certain convolutions highlight particular kinds of spatial relationships between pixels. Think, for example, of contrast, edges, curves, straight, or diagonal lines in images. Consequently, convolutions are able to automatically detect local patterns.{21}
A CNN’s architecture consists of multiple layers of convolutions that highlight particular low-level features. By combining the input of several of these layers, we can train the CNN: it learns that particular combinations of low-level features point to a specific feature of a cat: for example, the particular shape of a cat’s face or its pointy ears. As a part of an industry challenge by ImageNet, computer scientists have created CNN’s that are extremely good at recognizing (recall) and correctly classifying (precision) images into one of the 1,000 categories. With an error rate of just under 5% in 2015, the winner of the challenge in 2015 outperformed humans for the first time.{22}
Style Transfer & GAN’s
It’s not only possible to analyse images using computer vision techniques, moreover it is used to generate completely new images through the use of style transfers or GAN’s (generative adversarial network).
Style transfer as a topic is fairly intuitive once visualised; take an image and imagine it with the stylistic features of a different image. For example, in the style of a famous painting or artist. Undoubtedly, Style Transfer epitomises a novel use of neural networks that has ebbed into the public domain, specifically through last year’s Facebook integrations.{23} Style transfer is an older technique but converted to a neural networks in 2015 with the publication of a Neural Algorithm of Artistic Style.{24}
Over the last three years, researchers have made astonishing progress in essentially reversing these networks. Through a handful of generative techniques, it is possible to feed images into a neural network and then ask for a brand-new image that resembles the ones it’s been shown. Generative AI has turned out to be remarkably good at imitating human creativity at superficial levels.{25}
A generative adversarial network consists of two neural networks: a generator that learns to produce some kind of data (such as images) and a discriminator that learns to distinguish “fake” data created by the generator from “real” data samples (such as photos taken in the real world). The generator and the discriminator have opposing training objectives: the discriminator’s goal is to accurately classify real and fake data; the generator’s goal is to produce fake data the discriminator can’t distinguish from real data.{26}
GANs get around this problem by reducing the amount of data needed to train deep learning algorithms. And they provide a unique way to train deep learning algorithms to create labelled data – images, in most cases – from existing data.{27}
Rather than train a single neural network to recognize pictures, researchers train two competing networks. Extending the cat example, a generator network tries to create pictures of fake cats that look like real cats. A discriminator network examines the cat pictures and tries to determine whether they are real or fake. {28}
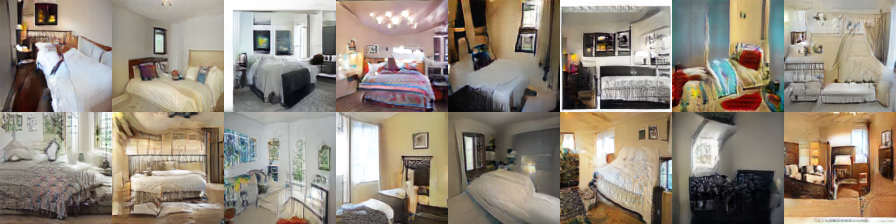
Moreover, when we let the GANS create new images of storefronts or interiors in our research it will also be able to tell us more where the computer vision puts emphasis on. In other words: the trial and error phase within this iterative process is important. The ‘failed’ designs by the computer are actually for us an important tool to get insight within out subject matter.
Possible research directions
At this moment we have to do more research, experiments and before we can come with a proposal for the final project. We want to use the coming months to more closely examine the following research directions and make tests to understand the technical feasibility of the different options.
• Definition of gentrification and the aesthetics that cohere with it
One of the challenging parts of this research is how we actually define gentrification and the aesthetics that cohere with it. Do we limit ourselves to the formal definitions and have to use socio-economic data before we can even look at which aesthetics would be connected to these processes? Another road might be to research how humans visually recognize gentrification on a personal level and how to teach a computer to see in ‘our’ way. As with any new technology, artificial intelligence reflects the bias of its creators. The formal and artistic documentation of this process is really relevant within the larger framework of the research.
• Image recognition and computer vision
At this moment there are different methods to use image recognition and computer vision techniques. This can range from ‘simple’ image object recognition (for example, recognizing the Dutch cargo bike, which is seen as a symbol of gentrification within the Netherlands, to recognizing the Canta, a car that is seen to be used by people with a more working-class background); but also think about parsing the captured footage of persons on the street to analyse their clothing. Within the research we are also focused on the recognition of visual styles and combinations of styles that are associated with gentrification. Is it possible to detect changes of colours in neighbourhoods? Do certain typefaces appear more often? Can we detect certain objects? And is there in general more variation in visual styles? Next to that the most interesting an unexplored area is that the computer can analyse the visual data and itself and might even come up with certain aspects we did not even notice while looking at the topic. For example, could there be a specific façade of the building structure that is used by bars/stores that could be classified as adapting gentrified aesthetical styles?
• GANS & Styletransfer
In relation to the development of the GANS and style transfer, can we use them to create generative street images and can we use them to forecast how a neighbourhood will develop in the future? Furthermore, even if our GANS are not perceived as real, is there information that can be dissected from it in the way the computer looks at our concept of gentrification?
• Scale
Furthermore, it is necessary to decide more specific on the scale of working, for example, building, street, neighbourhood and/or whole cities. As we might be operating in a global context we might have to develop ways of ‘crowdsourcing’ to get enough data.
• Stages of gentrification
Because Google Streetview documents the images for each year we can go back in time to see how neighbourhoods have become gentrified and if it’s possible to dissect different stages within this process. Instead of a binary question, it becomes a scale with different options.
• Globalising aesthetics
One of the possible directions is to research if it is correct as some scholars say there is an emergency of globalised styles. Will the software we make for Amsterdam also work for Seoul? And what would the errors say about national or local identities? Could we create visual ‘summaries’ of different (international) cities or neighbourhoods?
• Combining image recognition with other data
Apart from using (socio-economic) data sets to decide in which ways streets are gentrified it could also be used to look for other sources. Think about using location pinned posts from platforms as Instagram or Facebook. But also think of if it’s possible to include the photos of interiors that are linked to the Google locations; and or mating it with open databases like FourSquare, Funda, and Airbnb.
• Critical thoughts and speculative futures
Next, to the practical use of the software it opens up a lot of critical questions. What does it mean Google is the owner of this specific archive? While noting the progress of computer vision software, what utopic and dystopic scenarios can be made within our research area?
Similar research
A lot of corporate and scientific research is already focused on using computer vision. An interesting reference for our project is the ‘Places Database’ by MIT that offers a ‘Scene Recognition Demo’. It analysis images on the type of environment (think of outdoor/indoor), scene categories, scene attributes, and gives an informative region for predicting the category (where the image shows in the form of a heatmap why the image is selected to be in a specific category).{30}
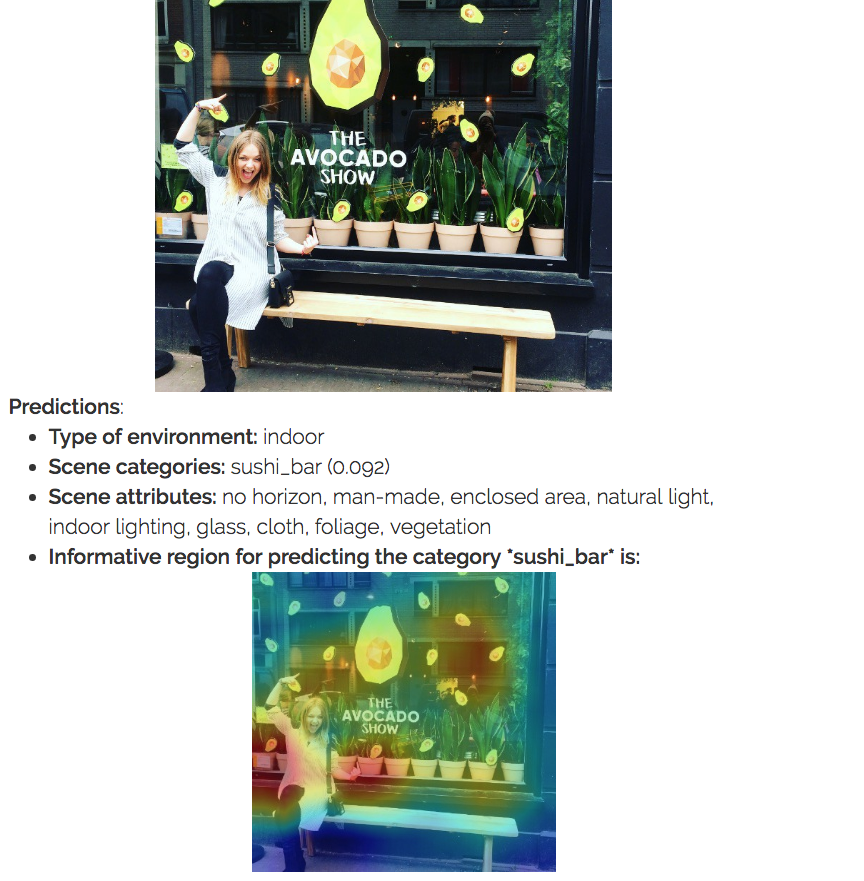
What’s relatively new about our research is to let the computer learn a concept that is a highly conceptual theme. However, there are already researchers that have worked on a similar topic. ‘Streetscore’ is a scientific project where a team of MIT scientists were “Predicting the Perceived Safety of One Million Streetscapes” and used Computer Vision to classify if a street would be perceived as safe.{31} This research is an interesting reference since it is not actually intending to research the actual safety of a neighbourhood, but rather a perceived safety, such in which the aesthetics of gentrification will always also be a ‘perceived’ state of it.
Playing with this ‘perceived’ state of gentrification is the research by Amsterdam-based studio Golfstromen. They used ‘crowdmapping’ as a way to get an insight in what inhabitants see as the border between gentrified parts of the city and non-gentrified parts. Taking the ‘average’ border out of many submissions, the research tried to predict the location of the ‘real’ border between gentrified and non-gentrified neighbourhoods in the cities.{32}
Relevance and goal of the research
There are several reasons why we want to research the Aesthetics of Exclusion. First and foremost, we have noticed a lot of changes within our own city (Amsterdam) that affect the way we live together in our neighbourhoods. It has become very easy to live totally segregated lives within one and the same city. The aspect that ‘gentrification is a process that hides the apparatus of domination from the dominant themselves’ draws our interest. In what way do these changes go ‘unnoticed’, while they might have a bigger effect on society that we are actually able to see. As part of the research, we hope to design systems that make it possible to show these hidden layers and interact with them. As designers we are responsible for how we are shaping our city and the aesthetics within it. This research aims to show this is not only a neutral position but also – without even noticing it - a very political one. By making it able to analyse these aesthetics, archiving it, making cross-sections, and creating ways to interact with it, we are opening up new ways of looking into the ways by which inhabitants are living together in urban areas.
Then there is a sincere curiosity in the rapidly evolving computer vision techniques. In general these are already having a big effect on our society --- something that will only increase in the future. In most instances market forces will drive the development of these techniques. This makes it important for the arts and sciences to also take position and fuel research that is led by other motives. We hope to achieve that we can show that computer vision can also be used as a tool to critically engage with societal developments.
Next to that there is a curiosity in research in what ways we can navigate, use, and distil from (growing) Google StreetView Archive. In the coming decades a new historical archive will emerge making it possible to find new ways to look at how cities change each year. It is not a coincidence that the scientists working on this project not only have an affinity with new technologies but also with the documentation of history.